The fast-paced development of Artificial Intelligence (AI) and embedded systems in recent years has changed how we perceive the future. From human implants to industrial automation to exploring deep spaces like the Internet of Things (IoT), Artificial Intelligence (AI), Machine Learning (ML), and their combinations are opening new doors to unexplored frontiers.
What Is Embedded Artificial Intelligence?
Embedded AI is defined as the capability of embedded resources-constrained devices that are usually used to effectively carry out tasks that demand human intellectual capacity. In other words, Embedded Artificial Intelligence is the application of AI algorithms and models at the device level that has the potential to function in isolation without the need for external intervention.
History of Embedded AI
Embedded Systems and AI have been around for a long time now. The success curves of Embedded AI have been quite different. AI failed to keep its promise to change the world for the better in the late 20th century because of the lack of low-cost and high-volume manufacturing of the demanded electronic hardware components. The lack of engineers and data scientists also played a vital role in the downfall of AI. On the other hand, the concept of Embedded Systems started gaining popularity in the 21st century. Today, ES and AI are counted among the technologies experiencing an innovation surge.
Is Artificial Intelligence Related To Embedded Systems?
An Artificial Intelligence system model will analyze the available data in order to make better business decisions. On the other hand, Embedded Systems are devices that can generate data or information through sensors that the AI algorithms can further use. The more trained models, the excellent the results.
On a broader level, AI and ES are connected. Embedded AI emerges and finds applications in autonomous vehicles, consumer electronics, and industrial sectors.
Why Should You Integrate Embedded AI Into Your Business?
For businesses, Embedded AI holds several advantages compared to conventional solutions. Here are a few benefits of Embedded AI that you can leverage for your business:
1. Reliability
A device that can process the data locally is less prone to failures and reduces downtime. This is the essential requirement for specialized tools in the industry, on which the users are highly dependent. Embedded AI Solutions are undoubtedly better than the conventional ones in terms of flexibility and reliability.
2. Latency
Embedded AI deployment dramatically decreased the latency of systems. It is simply because the sensor data is being processed locally instead of transferred to a secondary location for computing. It is essential for real-world services and applications requiring real-time solutions with Artificial Intelligence.
3. Privacy
Recording devices and sensors generate sensitive data and pose privacy concerns. Sharing this sensitive and confidential data over the multiple layers of the world wide web enhances the risk of privacy breaches. Hence, processing the data at the edge avoids data transmissions to reduce the probability of violation. Thus improving the privacy control of the devices.
4. Bandwidth
AI algorithms need a large amount of data to train and analyze the models. Bandwidth is required to transfer the data for computing to the cloud or other data centers. With embedded AI, devices become independent, and there could be little to no bandwidth for any device to perform its essential functions effortlessly.
5. Economics
Apart from the costs involved for any additional operations once the data reaches the cloud, deployment of Embedded AI solutions eliminates the requirement for communication with the cloud as the device can process the data and has the intense computing power to train the AI models, reducing the costs significantly.
Zaident assists elite clients in two steps to achieve innovation and create disruptive breakthrough solutions. The first is by moving the on-premise applications to the cloud. The second is to apply Artificial Intelligence. Machine Learning (AI/ML) models and algorithms to the transactional data to provide operational insights that would allow the client to drive cost reduction and improve revenue through better decision-making.
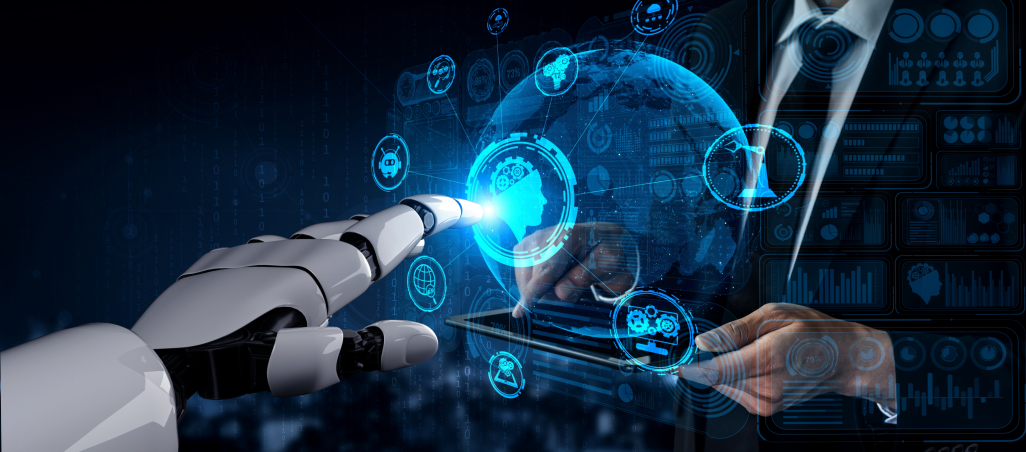